This is a draft version! Do not share the link externally!
Why the Design of AI Solutions Needs to Follow a Human-Centered Approach
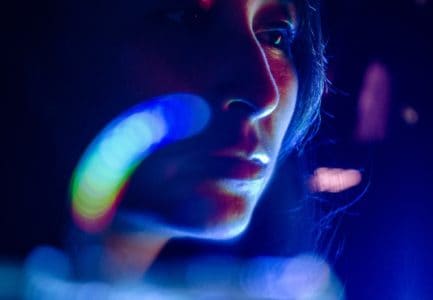
Artificial Intelligence (AI) is becoming more and more commonplace. While this offers huge opportunities, it also creates challenges of its own. That is because many end users have difficulties comprehending AI in all its aspects. As this is potentially limiting the technologies utility in the long term, modern solutions in this field need to adopt a human-centered approach by creating trust, fostering the understanding of capabilities and limitations as well as being transparent and open to feedback.
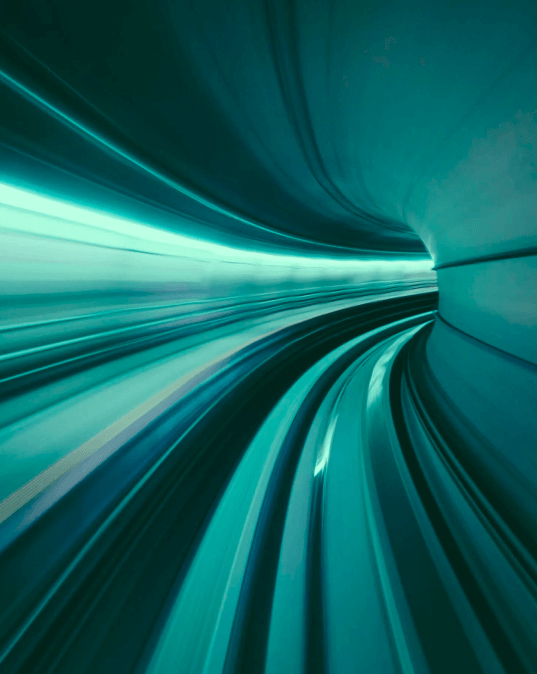
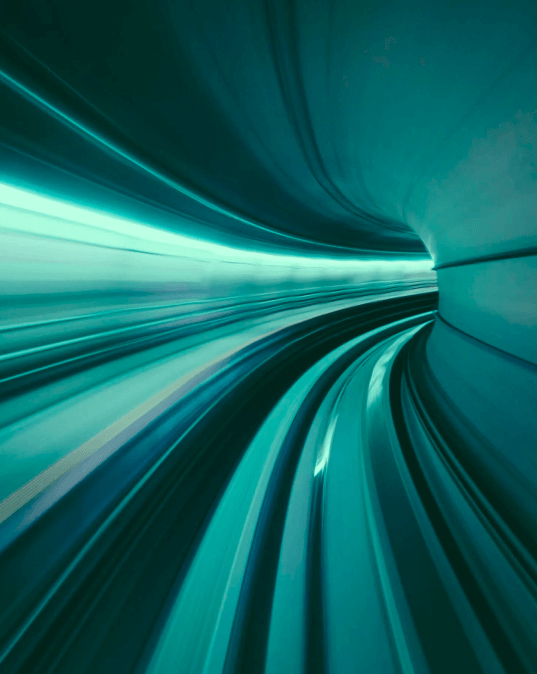
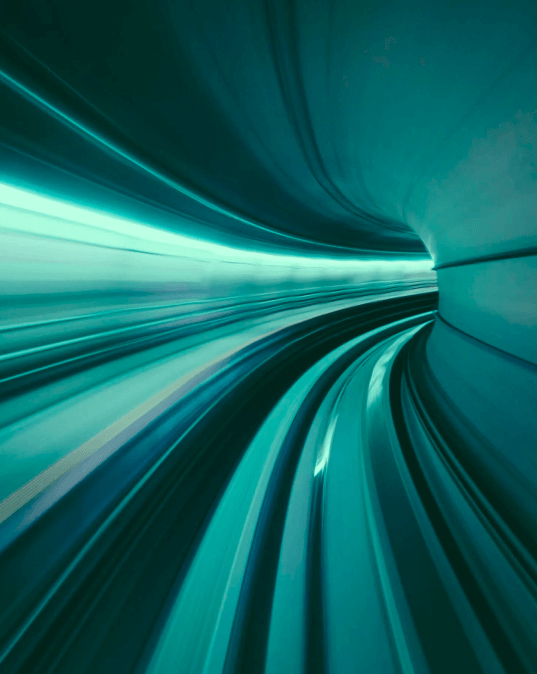
The first two waves of the AI evolution focused mainly on technological solutions in a straightforward manner, without considering other aspects, to achieve the desired outcome. Suffice it to say that this paved the way for the current third wave, characterized by major breakthroughs in the application of deep learning for big data, pattern and speech recognition. Consequently, the number of fully operational AI applications is rising rapidly, as is the number of industrial fields using them. Use cases span from the diagnosis of diseases in the health domain to supporting risk management decisions of finance industry employees. From this outset, it becomes clear that smart solutions utilized in the decision-making process offer huge opportunities. However, it also highlights one major challenge: Designing AI solutions that are better understood by humans and take the specific needs of the end-user into account.
‍

Novel approaches to designing AI solutions
This results in an ongoing discussion about how to combine technological advances with approaches such as human-centered artificial intelligences. Thus, current research projects focus on principles and frameworks that highlight aspects on how to design such systems. These novel approaches aim to help UX designers create an experience where human stakeholders such as users, operators and clients become part of a larger ecosystem. Long-term trust must be established among these stakeholders by matching the AI solution with their personality traits, as this is one of the key elements for any successful AI solution. To achieve that, certain needs have to be met.
‍
“Long term trust is one of the key elements for any successful AI solution!”
Dr. Martin Boeckle, Lead Strategic Designer at BCG X
‍
Getting rid of the black box
Every human-centered approach to AI solutions needs to overcome the existing problems in the relationship between human users and the algorithm. The biggest issue is the so-called “Black-Box problem”. This means that end users lack understanding of the underlying logic of a solution, leading to questions such as “Why did you do this?”, “Why is this the result?” or “Why not come to this and that conclusion?”. Since Machine Learning models are non-intuitive and very difficult to understand for different types of users, explanations will be needed. This is especially important for applications supporting critical decision-making processes.
‍

‍
Explainable AI as a key principle
Enabling users to answer the aforementioned questions on their own is a huge step in the right direction to create trust between humans and the AI solution and overcoming the black box problem. A whole research stream has dedicated its work to this goal, called explainable AI (XAI). In a broad sense, the communication of the specific systems limitations also falls under that purview. The end-users need to know to what extent they can trust the results put out by the system, and in turn when they should put them into question or even ignore them altogether.
‍
On the system side, many applications suffer from a lack of knowledge about the end-user needs. Since end-users never explicitly state what they require, XAI Systems must be ready to accept a mix of different types of information with the goal of adapting to changing capabilities and demands of their users.
‍
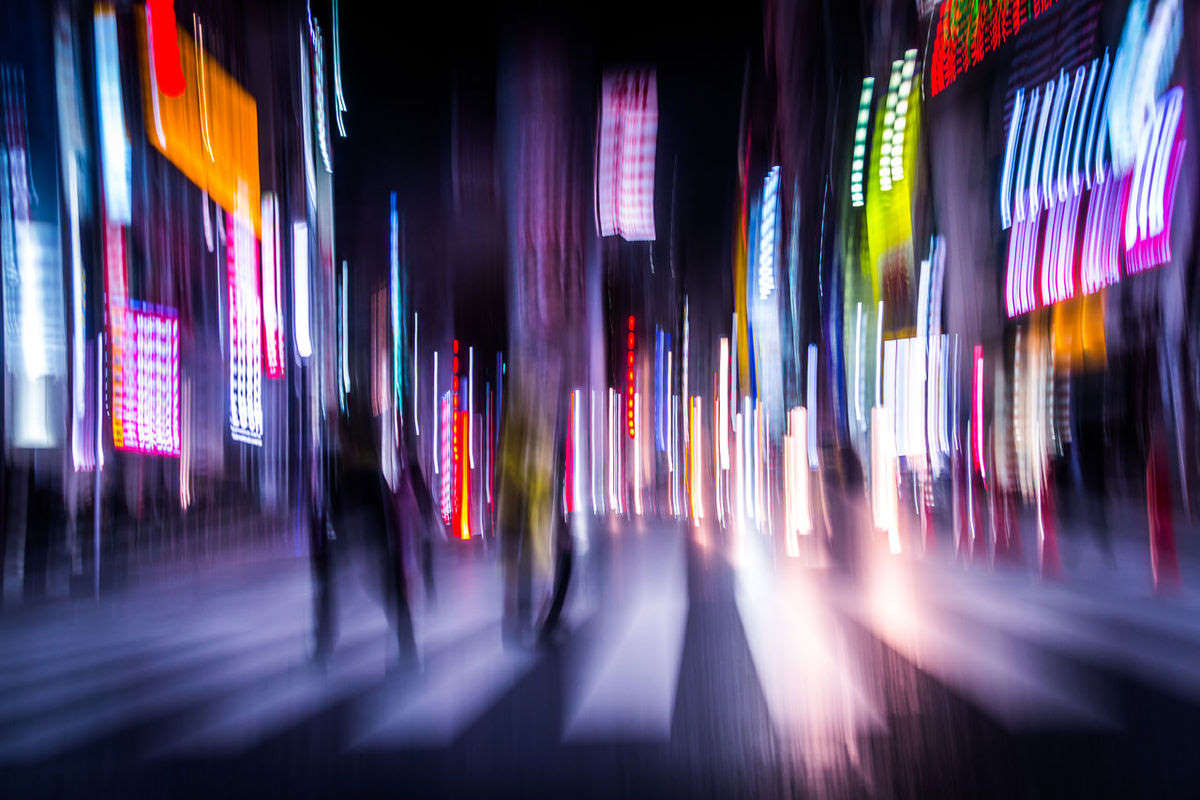
‍
A first structured approach to human-centered AI
So far, there is no commonly accepted structured approach to designing AI applications in a human-centered way. To change that, we propose a three-stage framework that highlights a mix of the traditional design thinking approach informed by an AI design process. This enables AI system designers to align their efforts with the end user requirements. Specifically, it consists of the following phases: Explore and define – AI Exploration, Ideate – AI Capabilities, Prototype and Test – AI Maturity
‍
"Our three-staged framework enables AI applications designers to develop their solutions in a human-centered way"
Dr. Iana Kouris, Associate Director at BCG X
‍
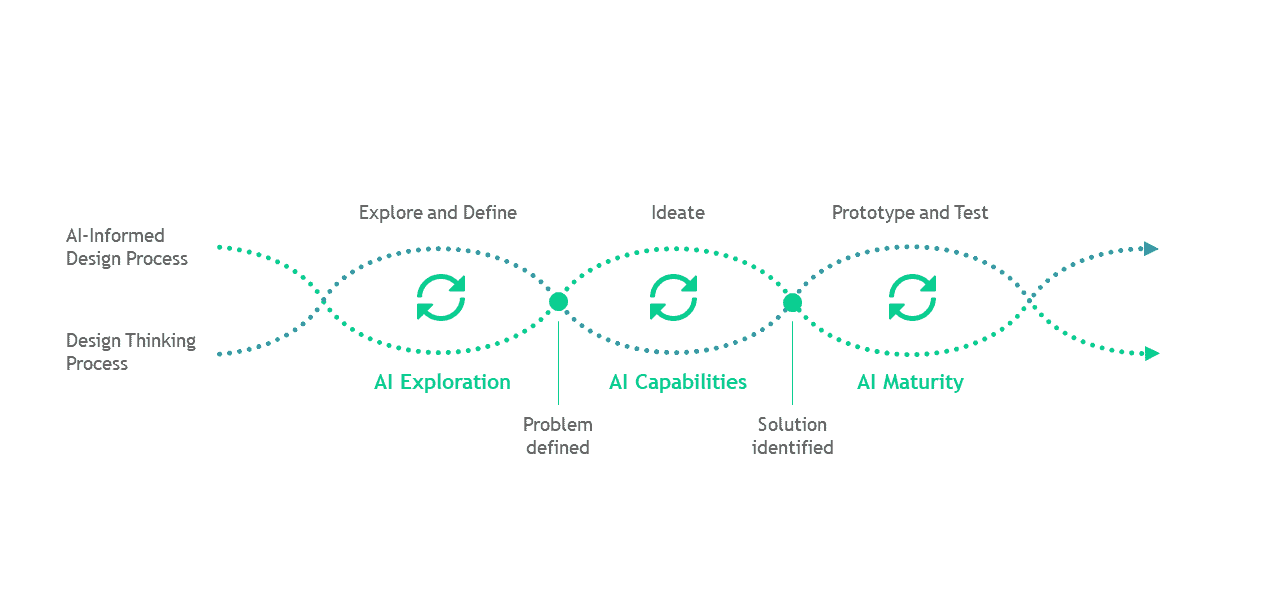
‍
Explore and define – AI Exploration
Identification of user needs is one of the most important steps in the design process of intelligent solutions. There is a large range of methods and tools available to uncover the end user needs, for instance surveys, expert interviews, ethnographic research, etc. Once known, an analysis of these requirements should yield valuable insights into which data will be needed as an end result of the AI solution and what quality said data must have. To get this right is of critical importance and a crucial point at the beginning of the AI exploration phase. When this is done right, the definition of point of views of the different stakeholders helps specify the overall problem statement and supports the ideation process in the later stage.
‍
Ideate – AI Capabilities
Potential ideas and solutions supported by AI capabilities should be discussed in the second phase. One of the main goals here is to identify intersections between the user’s requirements and the strengths of AI, followed by the translation of user needs into data needs. The data should also address various user groups, since a heterogenous group of end users will interact with the application. In order to identify the most relevant idea, the calculation of the real added value of an AI application should be incorporated. Potential solutions are being ranked based on desirability, feasibility and viability to determine the sweet spot for innovation with a specific focus on AI. The most important element in the evaluation process is to identify if the AI elements render a real benefit to the end user and if they solve the actual problem in the first place. Concurrently a decision must be made about which processes should be automated and which should only be augmented. Finally, it is necessary to address the question “What does success look like” to define the desired outcome of the project and appraise if the proposed concept has a realistic chance of creating value.
‍
Prototype and Test – AI Maturity
This phase covers building and deploying models as well the definition of the UX of the developed AI prototype, where certain challenges need to be addressed. By looking at the customer experience lifecycle, best practices for the UX design of such solutions ensure a meaningful user experience. To provide an overview, general design principles include definition of the reward function in order to understand what success or failure might look like.
‍
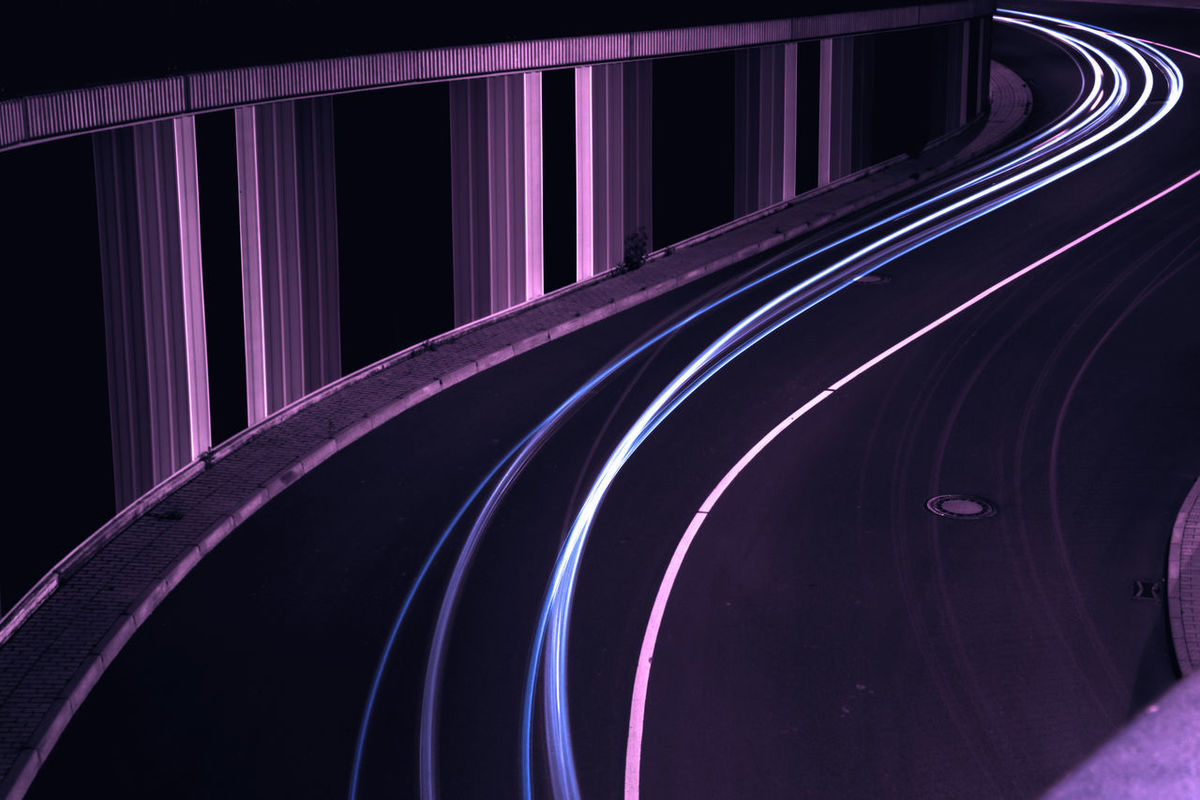
‍
The easiest way to build trust is by ensuring that the application provides correct and useful information right from the start, in line with its capabilities, limitations and the outlined expectations. Thus, within the onboarding phase “Setting the right point in time at which AI functionalities are being introduced” is one of the key principles.
‍
While interacting with the AI solutions the UX design should consider how to “Move forward from errors as well as how to reveal the source of the failure” by “Keeping the end users involved in order to learn from them through specific participation options and regular feedback”.
‍
The design of the explanation should always keep in mind that one explanation does not fit all. Thus, the personality traits of the end user should be considered at any time.
‍
As a result, current classifications discuss questions like “What is explained? (e.g. model or data)” as well as “How is it explained? (e.g. direct/post-hoc, static or interactive)”. Furthermore, designing for different outcomes is very critical as outputs need to be presented in different ways to reflect the confidence in the value.
‍

‍
Testing AI solutions goes hand in hand with building trust over time. Initial trust stems from the preliminary acceptance of the technology. Continuous trust builds over time and relies on the users own evaluation of the performance, reliability and security of the technology. While testing the AI maturity, one of the goals is to ensure the development and transformation from initial trust to a long-term trust building process.
‍
When testing and evaluating the AI applications, one needs to bear in mind that AI functionalities normally do not show their full potential at the beginning of usage. Due to this inherent feature, any evaluation needs to consider several stages with pre-determined value figures regarding capabilities that need to be checked against the real output. We recommend qualitative evaluation through interviews as well as data driven approaches where the quality of the customer experience lifecycle is being analyzed.
‍
"Design-centric corporations have about five times more loyal customers than those neglecting this aspect."
Dr. Martin Boeckle, Lead Strategic Designer at BCG X
‍
Human-centered AI – is it worthwhile?
The impact of utilizing a human-centered approach to AI is palpable and the figures can be compared to design-centric companies. Their benefits have been reliably evaluated, showing that they innovate and adapt faster and reap financial as well as human benefits. For example, design-centric corporations have about five times more loyal customers than those neglecting this aspect. They also display stock prices about 30 percent above their competitors and are four to five times more likely to have happy employees.
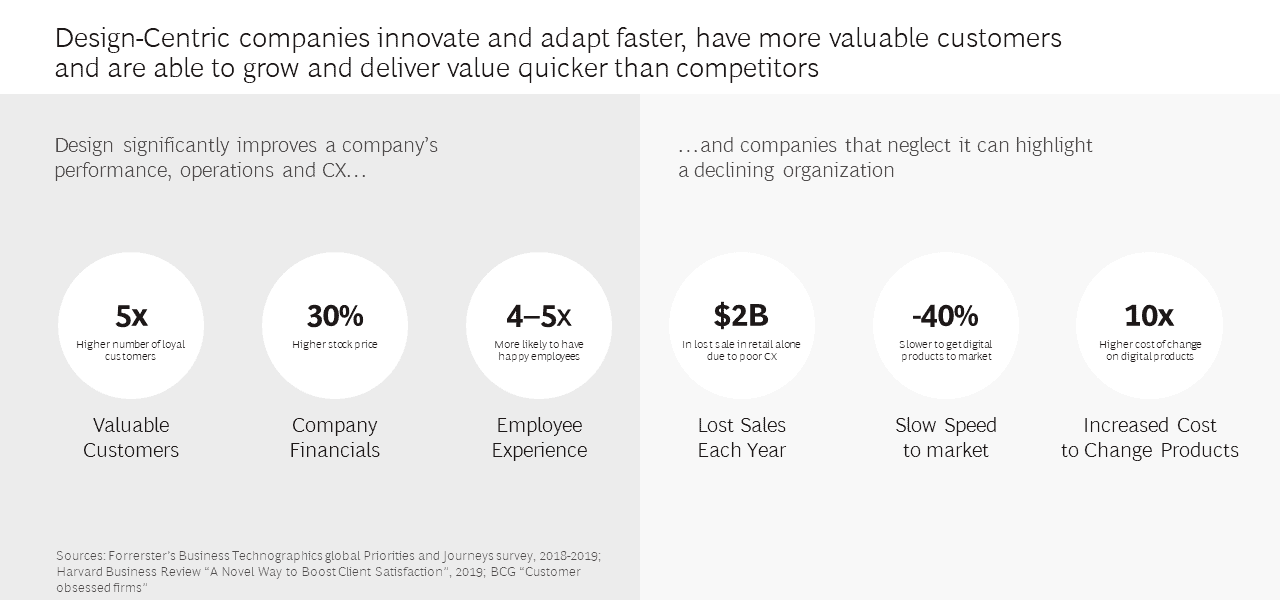
‍
Generally speaking, focus on user experience improves customer satisfaction, loyalty and overall customer engagement. Since a good UX normally leads to greater transparency of procedures and easier to use and understand products, the trust in the company is enhanced and easy accessibility is ensured. Therefore, while it might be easier to just generate AI technology for the sake of it, going the extra mile with a human-centered approach to AI is quite worth the while.