Insurers Realize Real-time Advantage by Doing Data Platform Dance
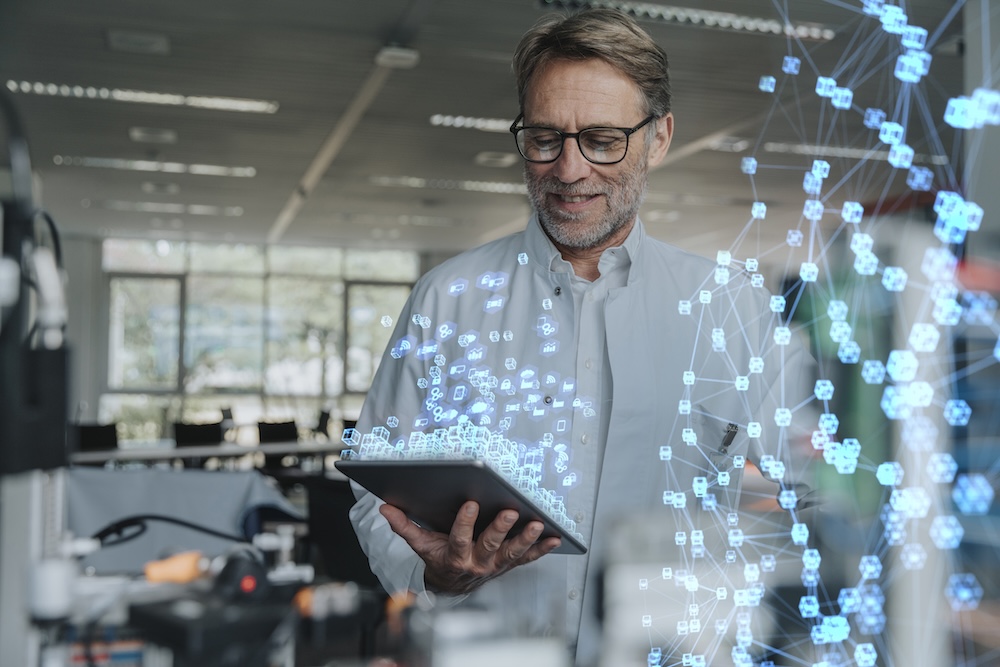
In the past few years, BCG Platinion has helped leading insurers in Europe and Asia to embrace a streaming-first data platform approach. This is where business events from sales, core insurance and cross-functional systems are streamed to, and processed by, a central data platform as they happen.
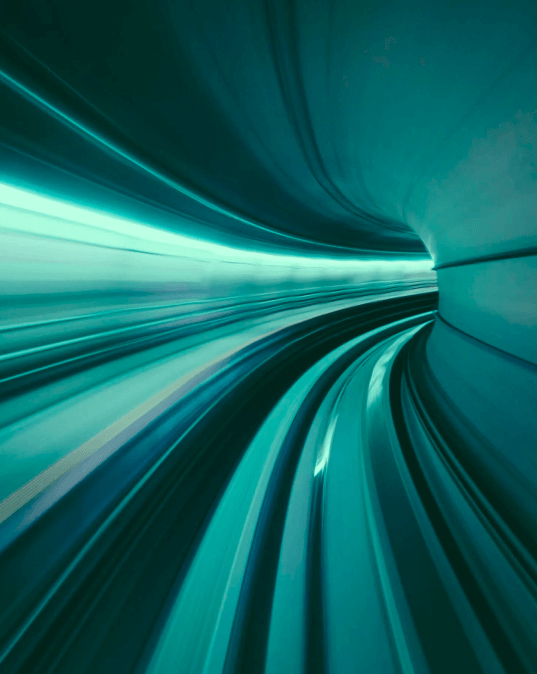
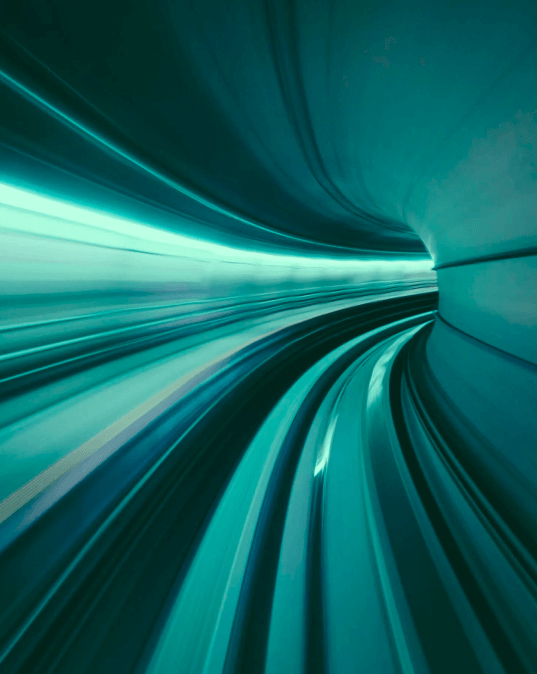
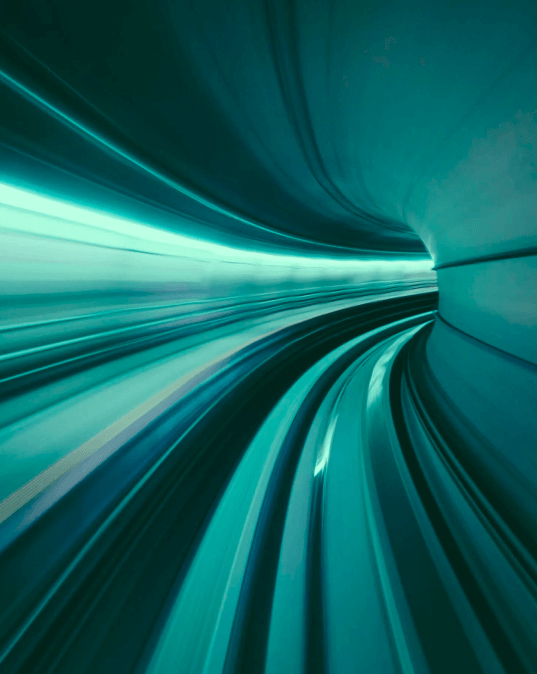
While this streaming approach is common in business domains like energy trading or financial market data provisioning - where there is a strong, real-time business model component - it remains fairly uncommon in areas like insurance. But the benefits are potentially transformational.
Imagine you are an insurer with a magic wand that enables you to see and work with every dataset in your business across the value chain, regions and lines of business in real-time. What could you do with it?
Here are two examples:
- Data-Centric Integration: you could display this data in your end user apps and across your processes in service centres, without having to request it from your cumbersome, slow and expensive core systems – and it would be “live”, not yesterday’s data.
- AI and GenAI: You could train and run your new AI models on the same data source– no need to integrate your models with complex operational data flows. Live data would be on the same platform as historical data, enabling you to adjust a price quote based on pretrained models and, if needed, even market data in real time.
While the ‘magic wand’ has been known about for years (CDC, see below), bringing a system like this into production has been challenging until now:
- There is a growing set of effective commercial and open-source tools available, with cloud platforms offering the scalability to support rapidly fluctuating data flows – supporting streaming-first methodologies.
- Data platform vendors are now providing more tools to support customers working with both streams of data, and tabular data sets, from the same source – supporting hybrid approaches.
So why do we think this is relevant to you?
A Paradigm Pirouette
Embracing an “everything-flows-data-architecture” paradigm means building the right data platforms to enable the “magic wand” mentioned above. Doing this calls for change data capture (CDC).

Once the ability to create a live copy of your core data is in place, challenges like access control and data optimization have to be overcome - but proven patterns can be used to do this. A real-time insurance dashboard can also be implemented to provide personalized data access based on the role of each user, ensuring data privacy while optimizing usability.
The method described above eliminates the need to establish parallel data architectures for real-time and historical data use cases, reducing technical complexity and enhancing overall efficiency. Data processing pipelines are often burdened by table reads or writes, which come from the perceived need to store data in tabular formats. Processing business events as they happen can simultaneously boost compute and storage efficiency.
Streaming Paradigm Stumbling Blocks
From a data engineering perspective, applying a streaming paradigm end-to-end is an advantageous prospect, but in reality, this route will present challenges for many insurers. Advanced skillsets are required to stay on top of core concepts and keep the tech stack up to date, and cost efficiency is difficult to get right – but there are other pitfalls to be aware of too.
Data users that are more familiar with SQL and tabular snapshot data may be at a disadvantage, requiring more time to complete a streaming paradigm shift. Business value extraction will be limited when technical platform evolution is prioritised above providing data users with familiar, easy-to-use data access patterns.
There are vendors and cloud service providers capable of helping players overcome these stumbling blocks:
- Cloud service providers offer services to connect to CDC tools, manage data streams, and provide tabular views of them.
- Data platform providers like Databricks and Snowflake, as well as specialized vendors likeRisingWave, offer similar capabilities.
RisingWave provides a tool for creating tabular views on top of data streams, while Databricks offers hybrid views via Delta Live Tables, and Snowflake via HybridTables. Each solution differs in its approach and characteristics, but the trend is clear: mature hybrid solutions now treat both tabular and streaming data in the same way. This is essential for helping teams familiar with SQL interfaces to transition (while still leveraging real-time data processing capabilities).
When using a streaming-first approach, data streams will need to be reprocessed – sometimes from the beginning. Repeated re-processing is costly and often introduces errors, but this can be avoided. The answer is maintaining materialized views of specific datasets, ones that are updated as events happen.
The solutions to most data streaming challenges are well understood, but applying them properly to production-grade setups requires specialized skills, as well as significant conceptual and engineering work.
The Hybrid Platform Waltz
We recommend setting up a hybrid platform capable of providing both data stream and dataset snapshots from the same source. The key is to take a two-step approach – first right, then left.
The first stage involves leveraging CDC and shifting data ownership to the ‘right,’ enabling data engineers and scientists to work on liberated datasets that are no longer hidden in the ‘data walls’ of cumbersome core systems. Doing this speeds up the creation of data products and real-time use cases, demonstrating the value of data to business stakeholders.
While this step to the right is crucial to“unfreeze” business data and prove its value, a second step is required to make this approach sustainable. The ‘left shift’ involves core system owners taking control of the business events they want to share with the central platform. If this step is carried out using a hybrid platform, streaming and snapshot capabilities can be leveraged depending on the dataset in question.
Essentially, the ‘left shift’ is Domain DriveDesign (DDD) – a methodology for developing complex systems. It works by separating domains by context, defining how they communicate via business events, and determining what data to share. The goal of this is to make operations more sustainable and systems easier to maintain.
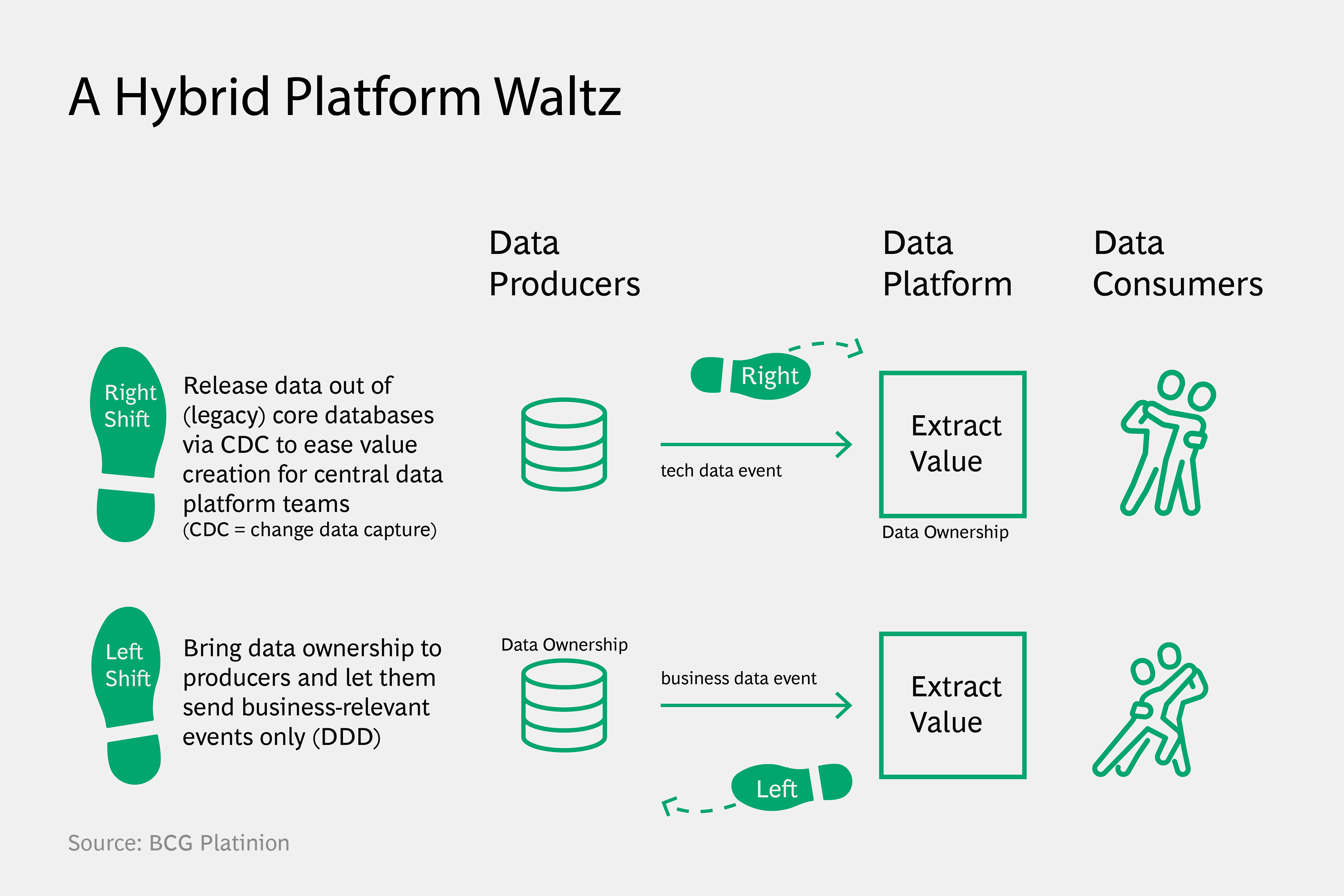
A hybrid platform of this kind will help address both data consumer acceptance and data ownership, providing known approaches when it comes to working with the key access data.
Data consumers will need to be involved and capable of informing the platform of what data they need and the form they need it in. An example of this could be establishing data contracts in KafkaStreams, enabling insurers to make certain that downstream users like actuarial teams only receive the relevant data. This boosts both consistency and quality as a result.
Because of this, the operating model of a streaming-first data platform is of the utmost importance, as it will support data workers and users alike as they operate in an end-to-end way. This should be supported by a self-service platform that automates data creation and consumption steps for data owners.
Hybrid solutions allow streaming-based data platforms to continuously view and update data in real-time tables. This approach combines easy-to-understand snapshot views of traditional tables, with the real-time data updates enabled by streaming data platforms.
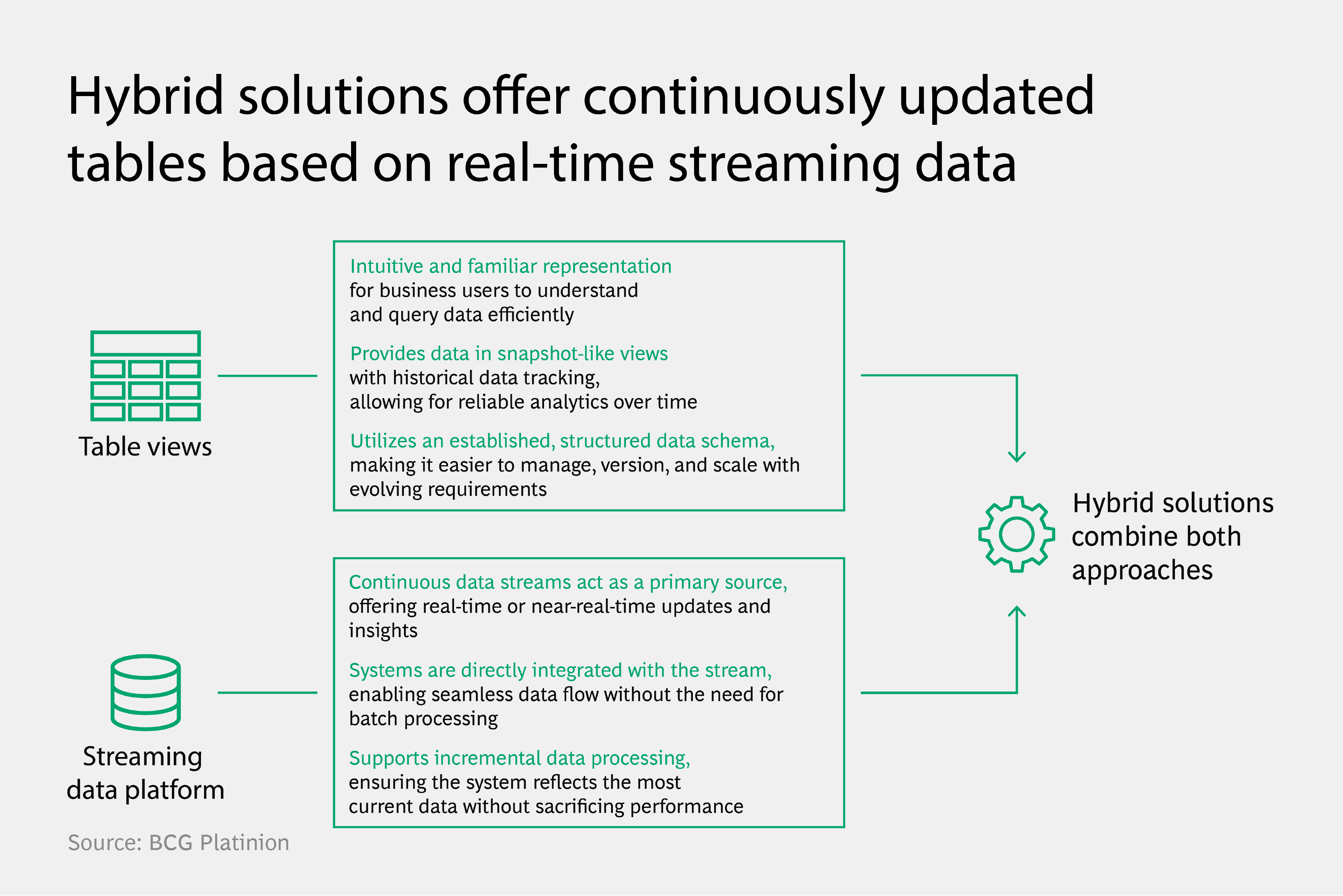
Choreographing Your Next Steps
Insurers need to have a strong understanding of data sources and platforms before starting the process of adopting a hybrid approach. First, you need to establish where you are in terms of untapped legacy data sources, and check the streaming readiness of existing platforms.
A gap analysis of business and IT demands focusing on live data access will provide a basis to work from. Begin by assessing which internal applications will benefit the most from real-time data, this process can be enhanced by using a proven data maturity framework - like BCG's own DACAMA framework. This will help you strategically select the right platforms based on scale, tooling maturity and cost structure.
Throughout this journey, it is critical tobe rigorous when it comes to value. While the idea of setting up an innovative platform and model can be appealing, players cannot afford to lose sight of fundamental business goals – setting and tracking KPIs remains essential.
If you have significant datasets locked up in legacy core systems and your data journey could be accelerated via CDC, or if streaming data is critical to meeting your business demands, the approach explored in this article could be a major breakthrough.
To get started on your large core systems, we recommend you build a team of data engineers capable of working with streaming data, and an initial op model setup that can support both key phases. Get in touch with us to find out more!